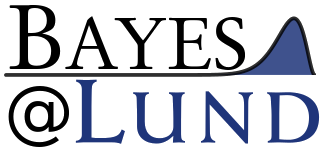
In April BECC hosted the half-day conference Bayes@Lund2014 with the purpose to bring together researchers at Lund University working with or interested in Bayesian methods. The conference was arranged by Ullrika Sahlin, researcher at Lund University Centre of Environmental and Climate research (CEC), and Rasmus Bååth, PhD-student in Cognitive Science.
Presenters from Ecology, Mathematical statistics, Philosophy, Statistics and Risk analysis gave their perspectives on how Bayesian methods are used in research at Lund University or what advantages Bayesian methods have over classical alternatives.
The first presenter was Johan Lindström from Mathematical Statistics who gave an excellent introduction to Bayesian and hierarchical modelling.
After than Yf Jiang, master student in Conservation biology, demonstrated specifying and running a Bayesian model in BUGS and R.
Yann Clough from the Centre of Environmental and Climate Research talked about the need to model causal relations and demonstrated a generalized approach to modeling and estimating indirect effects in ecology, which at the end required a Bayesian touch to get it to work.
Dragi Anevski from Mathematical Statistics brought up how Bayesian theorems can be useful in finding good estimators for parameters informed by data with certain characteristics such as resting time for migrating birds and x-ray time variability of galaxies.
Rasmus Bååth, Cognitive Science, rescued the mid-conference crisis by presenting his work on a R-package named Bayesian First Aid with the aim of Replacing null hypothesis tests by Bayesian estimation.
An interesting perspective on the controversy with the Bayesian approach, non-observed variables, and analysis of long term data were given by Krzysztof Podgorski from Statistics.
Ullrika Sahlin, from the Centre of Environmental and Climate Research, made an attempt to sharpen the arguments by providing several reasons to be Bayesian.
Finally, Martin Stjernman from Biology shared his concern of being a Bayesian wannabe in a presentation about incorporating uncertainty when evaluating subsidy effects on farmland bird biodiversity.
The vivid final discussion brought up experiences of the acceptance of Bayesian methods in research and the diminished role these are given in education compared to classical statistics. We discussed how to encourage the use and teaching of Bayesian methods at Lund University.
Bayesian statistics can be easier to understand than statistics relying on having many repeated observations (i.e. the classical) – it is designed to address uncertainty, is suitable for integrated modelling, it is able to deal with both expert judgment and empirical data, it uses probability in a way that is close to how decision makers interpret probability, and, if we allow ourselves to be subjective, it is more fun.
This event was highly appreciated and attended by about 55 participants from the Faculties of Science, Engineering, Humanities and Medicine. We decided to aim for a repetition in 2015.
For those of you interested to join a network of researchers and students interested in Bayesian methods there is a possibility to subscribe to an email list www.lucs.lu.se/bayes/.
Rasmus Bååth presented Bayesian First Aid, a project with the aim of making it easier to start doing Bayesian data analysis.
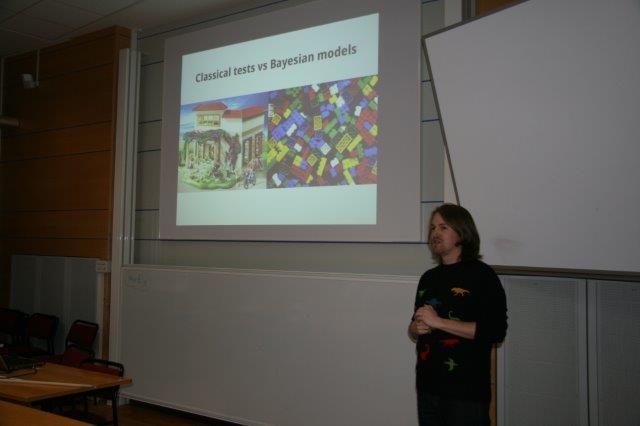
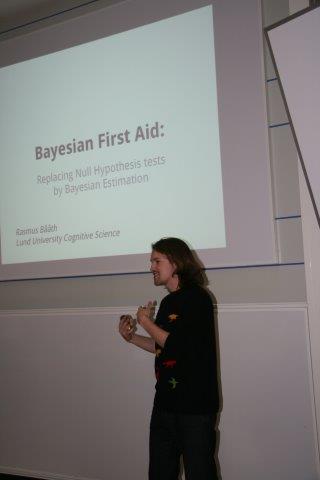
A multidisciplinary crowd attended the conferences with participants from biology, psychology, economy, mathematics, and cognitive science, among others.
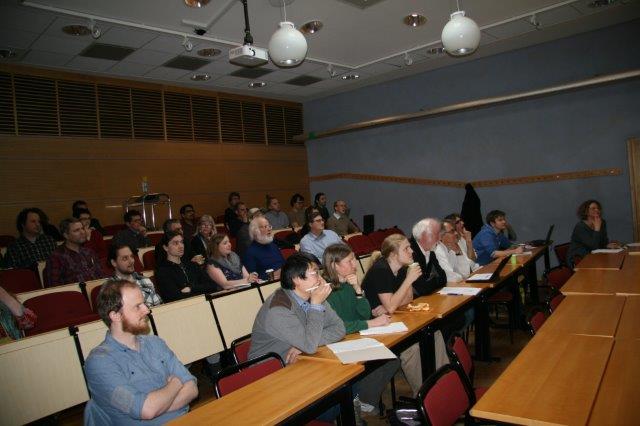
The discussions went on in the Fika break.
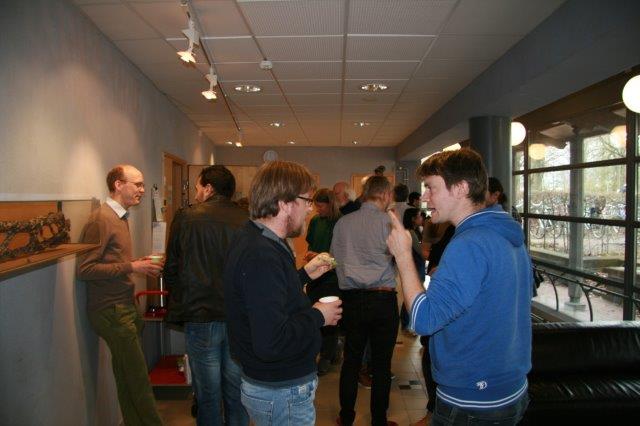
Comments